Keyword [MRI]
Yu L, Yang X, Chen H, et al. Volumetric ConvNets with Mixed Residual Connections for Automated Prostate Segmentation from 3D MR Images[C]//AAAI. 2017: 66-72
1. Overview
目前prostate MRI segmentation存在一些挑战
- 不同MR扫描协议导致MRI存在差别
- prostate MRI缺少清晰边界(prostate与周围组织很相似)
- 不同病变或分辨率导致prostate形状大小变化很大
- 3D MRI segmentation 耗时
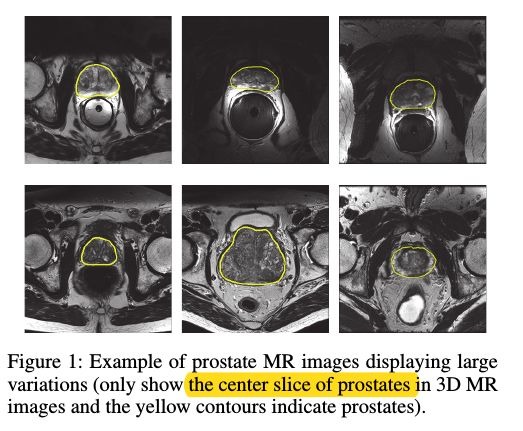
因此,论文提出一种end-to-end train, volume-to-volume predict, 适用于limited training data的模型,并在MICCAI PROMISE12数据集上达到state-of-art结果。
1.1. 模型特点
- 3D CNN
- 存在down sampling和up sampling两个阶段
- residual block和connection. block内部存在short connection, block之间存在long connection(恢复down sampling造成的空间信息丢失). 能够增强局部信息和全局信息在整个网格中的传递,以及加快收敛,避免梯度问题
- 加权(0.3, 0.6, 1) auxiliary cross-entropy loss. 加快收敛; Function as a strong regularization which is important for limited training data
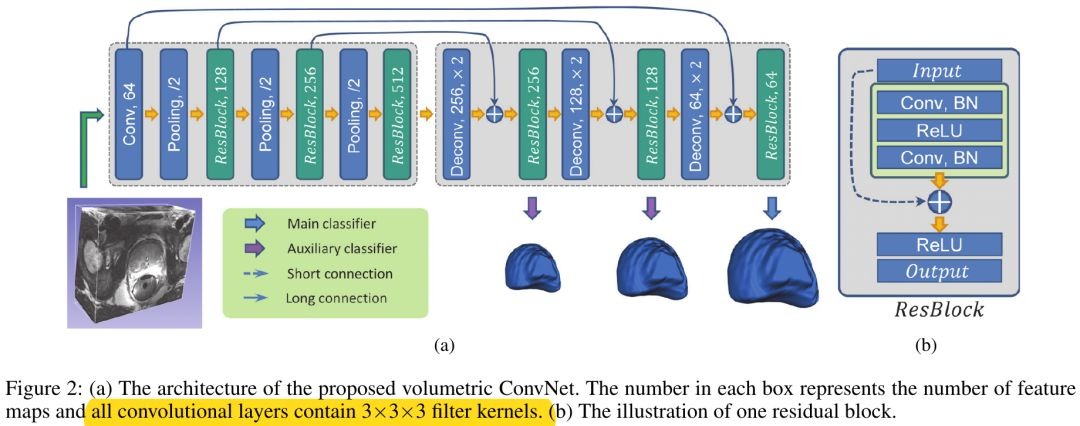
1.2. Dig the Potential of limited data
- Data augmentation. 但增加的信息量有限
- 使用skip connection. 提升网络内信息的propagation
1.3. Related Work
Multi-altas based method
- Deformable method
- Graph cut based mathod
- Feature based machine learning method(Deep Learning)
- 使用2D CNN aggregate 3D contextual feature. adjacent slices, orthogonal planes, multi-view planes. 但没有充分利用3D空间信息
- 3D CNN. with residual block
1.4. 数据集
MICCAI PROMISE12
- 训练集. 50 transversal T2-weighted MRI and segmentation gt
- 测试集. 30 MRI, 未提供gt
图像来自不同医院、设备、协议以及存在maximum variation in clinical setting (voxel size, dynamic range,position, field of view and anatomic appearance.).
2. Experiments
2.1. 数据预处理
- resize MR volumes into 0.625x0.625x1.5 mm
- 归一化
- rotation (90°, 180°, 270°) , x-flip
2.2. 训练细节
- batch size 8
- lr 0.001 divided by 10 every 3000 iteration
- weight decay 0.0005
- SGD, 0.9 momentum
- weighted loss (0.3, 0.6, 1)
- 训练(4小时). random crop 64x64x16 sub-volume
- 测试(12 s/MRI, 320x320x60). overlap sliding window (64x64x16), stride (50x50x12), average probability map
2.3. 评价指标
- Dice coefficient (DSC). 链接

- aRVD. absolute difference between volumes
- ABD. average over shortest distance between the boundary points of volumes
- 95HD (95% Hausdorff distance). 链接

2.4. Ablation

2.5. 实验结果
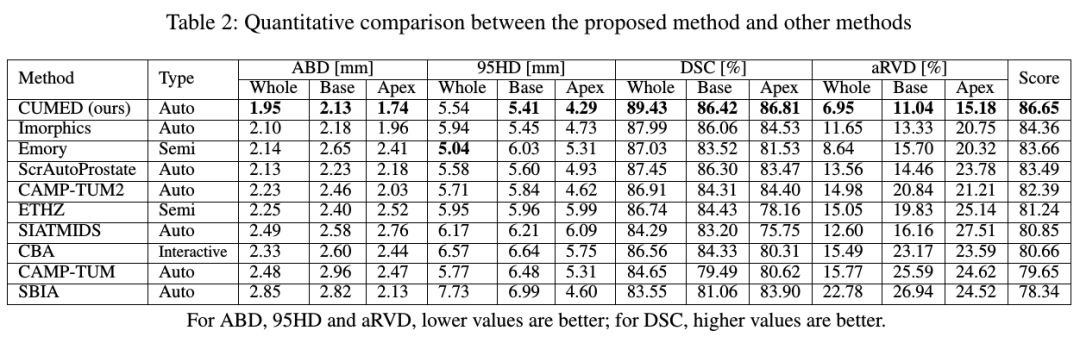
